Skeleton Tracking With Deep Learning - Loopy vs. Centipedes
Written on Wednesday April 14, 2021
Researching motion patterns can be time-consuming, especially if you have to track a lot of similar-looking body parts like the segments of worms, the spine of a fish or, what I show below, the tergits of centipedes manually.
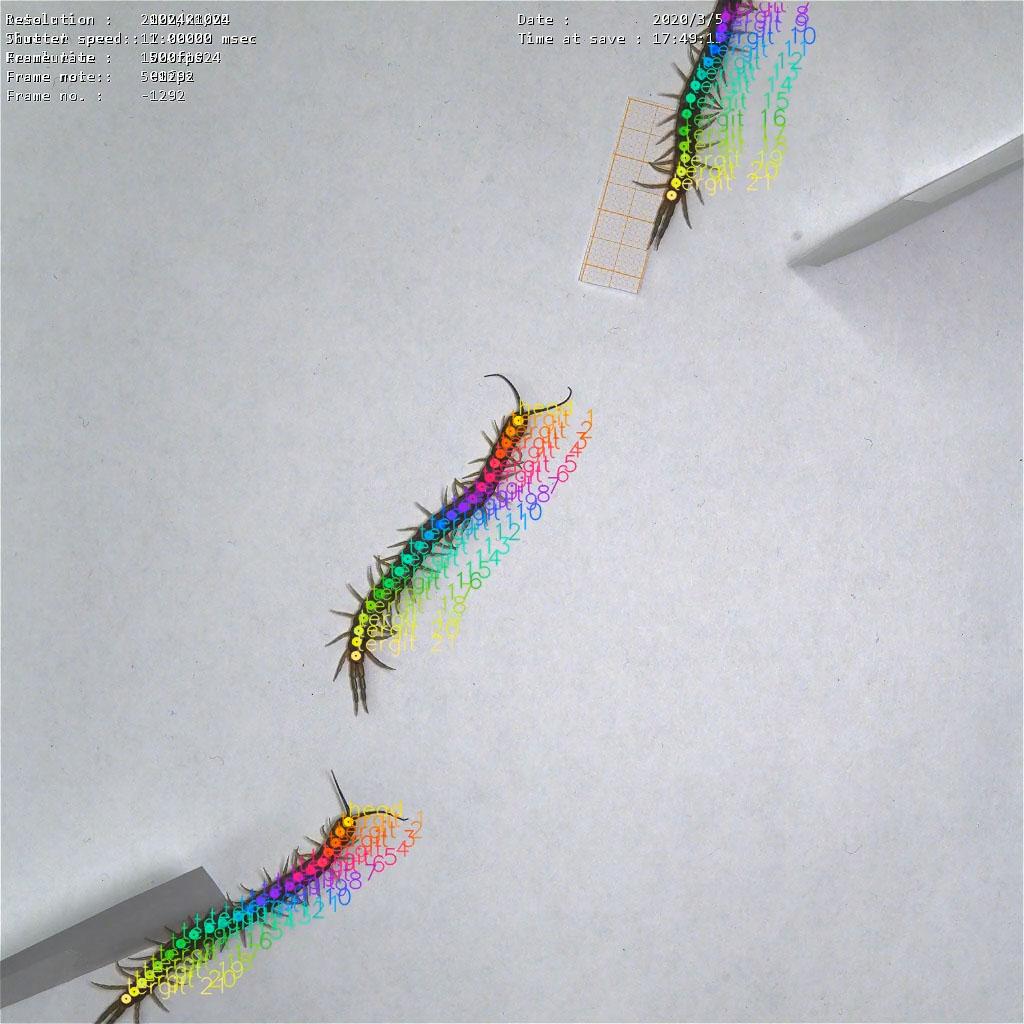
Multiple skeleton-tracked Scolopendra oraniensis.
Luckily there is a way to overcome these problems. Using the deep learning-based Keypoint detector from Loopy saves a lot of time and energy by not having to manually track the animal or body parts frame by frame. You manually annotate the points you are interested in manually in a few images and Loopy will train an optimal model for you - you don’t need expensive hardware or any programming skill for that as all is happening on the Loopy server. The resulting model will locate the animal or the different body parts, depending on your research question. Sign up for a free trial for Loopy today and feel free reaching out to me if you have any questions (christina@loopbio.com).
In parallel to my work at Loopbio I’m working as a member of a research team (Valentin Blüml, MSc; Christina Kaurin, MSc; Dr. Andy Sombke) at the University of Vienna. We are trying to discover the underlying mechanisms of myriapod locomotion in the Scolopendromorph, Scolopendra oraniensis, by using Loopy. Our main interest lies in the correlations between body undulation and speed, as well as the function and movement of specific legs.
There are few old studies describing the locomotive gait of Scolopendromorphs. Methodically these are limited due to the lack of high-speed cinematography and modern data analysis techniques available when they were performed. However, in the past years, the boom in AI technology and improved video recording hardware has made the advanced analysis of myriapods locomotion feasible.
Manual video tracking would have been an insurmountable amount of work but new machine learning technologies, such as those provided in Loopy, make tracking of segments, legs and antennae possible within days or hours.
A little bit of self-promotion: The advantage of Loopy compared to other toolkits out there is that you use it from within your browser. You don’t need to instal anything or have fancy hardware, all the heavy lifting is done on our servers. Sign up for a free trial , upload a video, start annotating a bit and train a model within minutes.
High Speed Locomotion
When moving at higher speed a greater body undulation should be visible as this would act to increase the step size.
To correlate body undulation and speed the head and 21 tergits of 14 individuals have been tracked with Loopy's deep learning-based pose-tracking module. Tracking manually would have been time-consuming and practically impossible, because of the amount of similar-looking body parts. After annotating some images I started training some models in parallel with permutations of the few training parameters. Loopy started to pick up the identity of the individual body segments reliably after about 300 images being annotated. To reduce false negatives and to increase the precision of detection I decided to annotate some more images by setting the markers at the center of the head and each tergit. The determination of any other position would have been way too difficult and thereby too imprecise.
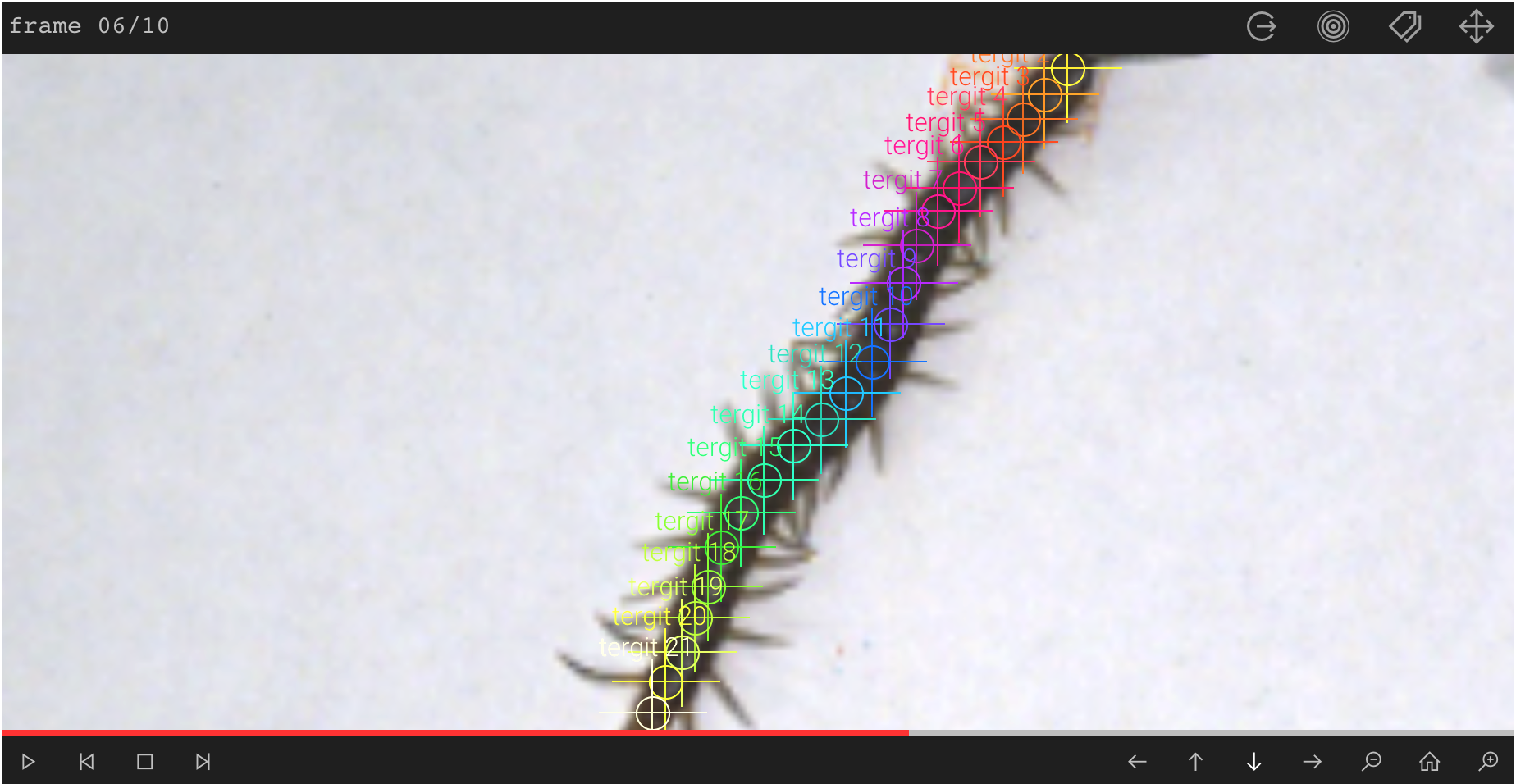
Annotating the individual tergits to train the skeleton tracking deep learning model.
Automatic tracking of individual segments by their identity works very reliably now. We are currently looking into the actual kinematic motion data and we hope to publish our findings soon.
Function and Movement of specific Legs
The last few leg pairs can be a bit special in terms of locomotion so we wanted to look at these with the help of keypoint tracking as well. In contrast to the running legs the last pair of legs (ultimate legs) is facing posterior and thereby does not participate in locomotion. In different taxa they may have different functions, like for example defense, to threaten possible predators, or to hold onto different surfaces, like the ceiling of a cave to catch prey.
Videos have shown that not only the last, but also the penultimate pair of legs often aren’t used for walking, but are dragged, especially when the animals are moving slowly. So perhaps there is a correlation between the movement of the 20th pair of legs and the speed of the locomotion.
To test these observations the last 3 pairs of legs and the antenna were tracked. Our study is far from being finished but we are confident that our hypothesis will hold.
Without Loopy it would have taken a significant amount of time to track each tergit, the antenna and the different pairs of legs if it would have been possible at all. Studies like this show that machine learning-based programs gain more and more importance in today’s research and thereby make the impossible possible.
Care to try Loopy yourself? By clicking here you can sign up for a free trial.